Computational Biology Hub
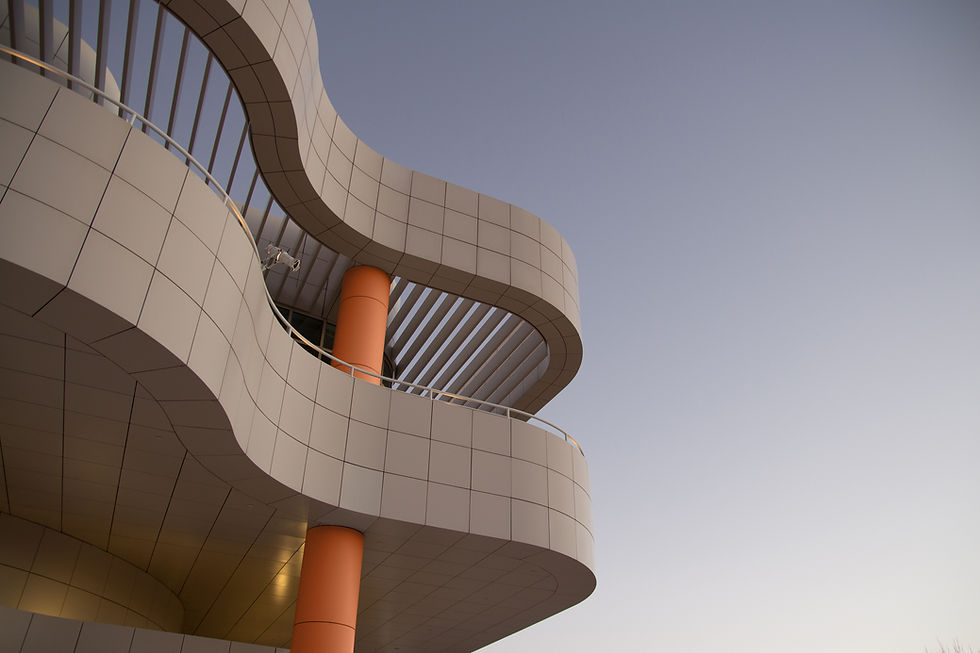
Decision Tree Regression Modeling for Beginners
Sep 18, 2024
2 min read
0
12
0
Are you interested in predictive modeling but feeling overwhelmed by complex algorithms? Decision tree regression might be the perfect starting point for you. In this blog post, we'll explore the basics of decision tree regression, its advantages, and how to implement it using Python.
What is Decision Tree Regression?
Decision tree regression is a machine learning technique used to predict continuous values based on one or more input features. It works by creating a tree-like model of decisions, where each internal node represents a "test" on an attribute, each branch represents the outcome of the test, and each leaf node represents a prediction value.
How Does It Work?
Tree Construction: The algorithm starts with the entire dataset and recursively splits it into smaller subsets.
Splitting Criteria: The splitting is based on which feature and value result in the largest reduction in variance of the target variable.
Stopping Criteria: The process continues until a predetermined stopping condition is met (e.g., maximum tree depth, minimum samples per leaf).
Prediction: To make a prediction, you traverse the tree based on the input features until you reach a leaf node.
Advantages of Decision Tree Regression
Easy to understand and interpret: The decision-making process is transparent and can be visualized.
Handles both numerical and categorical data: No need for extensive data preprocessing.
Requires little data preparation: No need for normalization or scaling of features.
Non-parametric: Can model non-linear relationships without assuming a specific functional form.
Implementing Decision Tree Regression in Python
The easiest way to implement Decision Tree Regression in Python would be to import DecisionTreeRegressor from the Scikit-learn library.
The import code should look something like this:
import numpy as np
from sklearn.tree import DecisionTreeRegressor
from sklearn.model_selection import train_test_split
from sklearn.metrics import mean_squared_error
import matplotlib.pyplot as plt
After importing DecisionTreeRegressor, import whatever biological dataset you would like. Define your X & Y variables, the train/test split, create and train the regressor, make the predictions, and visualize/interpret the results.
Decision tree regression is an excellent starting point for those new to machine learning and predictive modeling. Its intuitive nature and ease of implementation make it a valuable tool in any computational biologist's toolkit. As you become more comfortable with this technique, you can explore more advanced topics like ensemble methods (e.g., Random Forests) that build upon the decision tree concept.
Remember, although decision trees are powerful, they can be prone to overfitting. Always validate your model's performance on unseen data and consider techniques like pruning or setting appropriate hyperparameters to improve generalization.