Computational Biology Hub
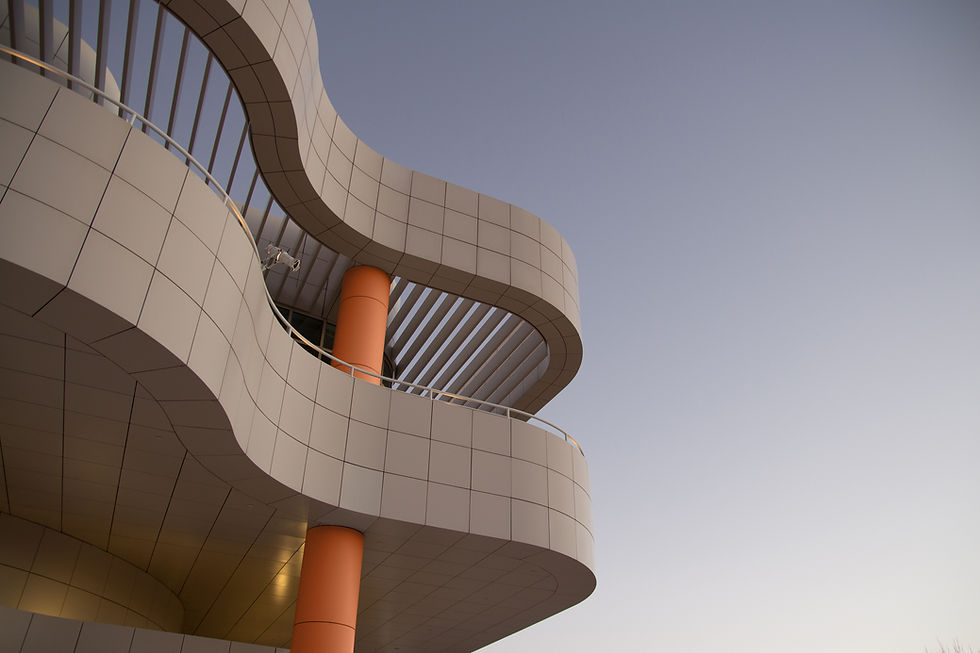
Bioinformatics Applications for Hidden Markov Models
Sep 18, 2024
3 min read
0
15
0
In the vast landscape of computational biology, Hidden Markov Models (HMMs) stand out as a powerful and versatile tool. These statistical models have found numerous applications in bioinformatics, helping researchers unravel the complexities of biological sequences. In this blog post, we'll explore what HMMs are and how they're applied in various areas of bioinformatics.
What are Hidden Markov Models?
Before diving into applications, let's briefly understand what HMMs are:
HMMs are statistical models that assume the system being modeled is a Markov process with hidden states.
They consist of two types of variables: hidden states and observable outputs.
The model transitions between hidden states based on probabilities, and each state emits observable outputs.
In bioinformatics, the hidden states often represent biological features, while the observable outputs are typically the DNA, RNA, or protein sequences we can measure.
Key Applications of HMMs in Bioinformatics
1. Gene Prediction
One of the most prominent applications of HMMs in bioinformatics is gene prediction. HMMs can be used to identify coding regions (exons) and non-coding regions (introns) in DNA sequences.
How it works: The hidden states represent different parts of a gene structure (e.g., exon, intron, intergenic region), while the observable outputs are the nucleotides in the DNA sequence.
Advantages: HMMs can capture the complex patterns of gene structure, including splice sites and start/stop codons.
2. Protein Family Classification
HMMs are excellent for modeling protein families and domains, helping to classify new protein sequences.
How it works: The hidden states represent positions in a multiple sequence alignment of a protein family, while the observable outputs are the amino acids in the sequence.
Tools: HMMER is a popular suite of programs that uses profile HMMs for sensitive database searches using statistical descriptions of sequence family consensus.
3. Sequence Alignment
HMMs provide a probabilistic framework for sequence alignment, both for pairwise and multiple sequence alignments.
How it works: The hidden states represent alignment columns, while the observable outputs are the characters in the sequences being aligned.
Advantages: HMM-based alignments can handle insertions and deletions more naturally than traditional alignment methods.
4. Protein Structure Prediction
HMMs can be used to predict secondary structure elements in proteins.
How it works: The hidden states represent different secondary structure elements (e.g., alpha-helix, beta-sheet, loop), while the observable outputs are the amino acid sequences.
Applications: This approach is often used as part of more complex protein structure prediction pipelines.
5. CpG Island Detection
HMMs are useful for identifying CpG islands, regions of DNA where CG dinucleotides occur at higher frequency than expected.
How it works: The hidden states represent CpG island and non-CpG island regions, while the observable outputs are the nucleotides in the DNA sequence.
Importance: CpG islands are often associated with gene regulatory regions, making their detection crucial for understanding gene expression.
6. Phylogenetic Analysis
HMMs can be used in phylogenetic analysis to model the evolution of sequences along a phylogenetic tree.
How it works: The hidden states represent ancestral sequences, while the observable outputs are the extant sequences we can measure.
Applications: This approach can be used for ancestral sequence reconstruction and to study the rates of evolution along different lineages.
Hidden Markov Models have proven to be an invaluable tool in the bioinformatician's toolkit. Their ability to capture the probabilistic nature of biological sequences makes them well-suited for a wide range of applications, from gene prediction to protein structure analysis.